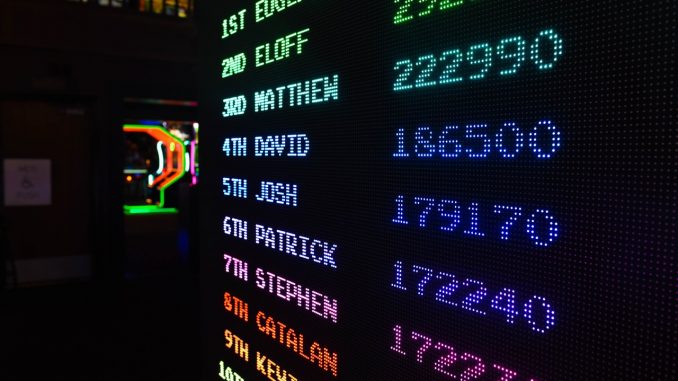
Whilst most companies use measures, key performance indicators and dashboards, they may work against the overall company goals.
KPI’s work in a similar way to a score track or health points etc in a game or simulation, offering visualisation of, how well a player is performing in the context of the game.
In most cases, a game or simulation needs a defined winning condition. If this is unclear to the participants, they cannot play to their best abilities and may become completely disengaged. Knowing the winning conditions will generally inform and influence any decisions taken during the game. This is dependent on two other points:
relevance – understanding one’s role in achieving the winning condition.
ability – being able to achieve those conditions.
This second point, ability, can also be affected by personal perception, believing one is able to achieve something irrespective of whether that belief is well founded.
So, it is in the real world. A lack of measures leaves the individual unsure of their progress or whether they are doing the right thing. Measures that don’t relate to your work-life or ones you feel ill-equipped to affect do not inspire and may demotivate. Visible measures that are achievable and understood inform and influence decisions. Where the importance of measures is increased through bonuses or other reward mechanism, they truly become the scoreboard of your working life and may disproportionately influence decisions towards an unanticipated and detrimental direction.
One difference between the simulated world and the real world is that in the real world the measures are not necessarily directly linked to a goal and the goal may not be clearly defined and articulated.
Exploring measures in game-based learning
The impact of measures/KPI’s can be usefully and practically explored in game-based learning by having different players or teams with different success criteria or winning conditions. The session facilitator can also investigate how the impact of these criteria differs when kept hidden or shared with the other players/teams.
Simulations can provide a very powerful tool in understanding the interplay of these elements and in transferring this insight back to the real world.
Using objectives in simulations
Objectives, or strategies can set rewards for interim achievements or end-game scoring. Below you see an example of the achievement cards I have for the Stabil-IT simulation. I have a few cards that can be placed next to the board to provide interim targets. The cards can also be used to introduce advanced gameplay avoiding the need for all the information to be given at the beginning of the game.

Here you see two alternative versions of the reward for solving incidents either setting it at the same level (2 points) or a higher level than the other achievement cards, as well as the common information on the back of the card (only revealed on achieving the objective). Focusing on the short-term objective can cause a player or team to lose site of the overall goal, even to the point of risking their ability to succeed. It can also place them in destructive competition with other players/teams. Using this type of mechanism in a simulation can help to create and reinforce awareness of the risk of short-term target driven rewards in achieving the long-term goal for the company.
- KPI’s – keeping score - 17th February 2020
I think that this is one of the most powerful applications of games in learning, particularly when using digital games. Tabletop games are brilliant for facilitating learning, but do not have the potential for easy large-scale data collection and analysis which can be implemented when using a digital medium. (Although they can be combined. I’ve had some great successes implementing a face-to-face experience with e.g. Excel, in the background doing the heavy lifting on the number-crunching)
Points are so often ‘just’ used to keep track of who is winning within the game, but the capability is there to create really nuanced feedback if performance criteria are carefully designed and linked to decisions made in the game. As an example, a digital game has the capability not only to track the results of a decision made in gameplay, but an enhanced capability (as compared to a tabletop game) to track dozens or even hundreds of datapoints relating to the process that led to that specific decision – leading to very granular learning.